Sentiment Analysis with pandas.apply: A Practical Use Case
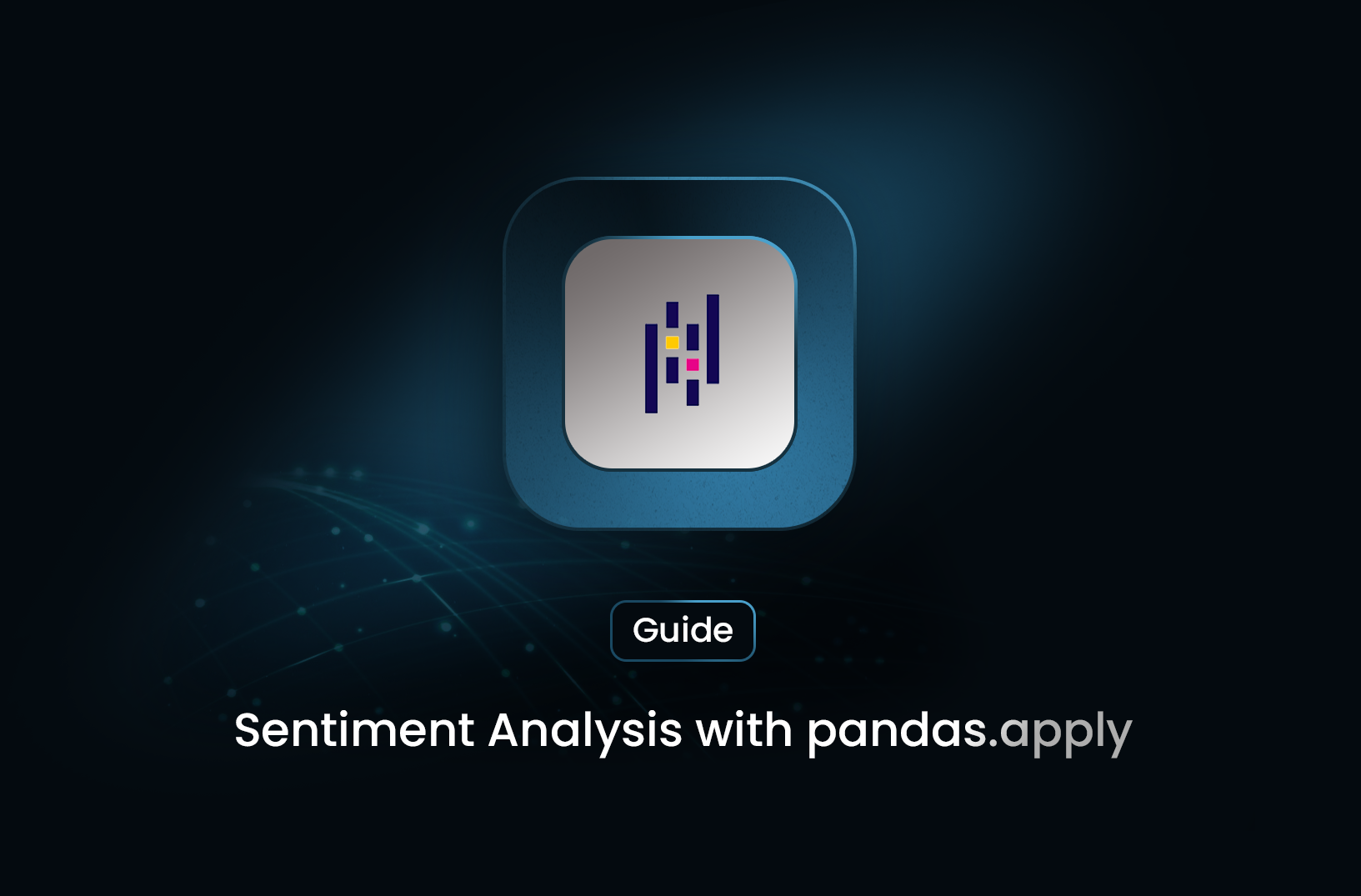
This guide demonstrates how to use pandas.apply
for sentiment analysis on customer reviews. We'll classify reviews as Positive, Negative, or Neutral using a custom function.
Problem Statement
You have a dataset of customer reviews and want to classify each review based on its sentiment using a polarity score.
Dataset Example
import pandas as pd
# Sample DataFrame
data = {'Review': [
"The product is fantastic, I love it!",
"Terrible experience, I will not buy again.",
"It's okay, but could be better."
]}
df = pd.DataFrame(data)
# Display the DataFrame
print(df)
Output:
Review
0 The product is fantastic, I love it!
1 Terrible experience, I will not buy again.
2 It's okay, but could be better.
Solution: Sentiment Classification with pandas.apply
Step 1: Install the Required Library
For this use case, we'll use the TextBlob library for sentiment analysis. Install it using pip if not already installed:
pip install textblob
Step 2: Define the Sentiment Analysis Function
from textblob import TextBlob
# Function to classify sentiment
def classify_sentiment(review):
analysis = TextBlob(review)
if analysis.sentiment.polarity > 0.2:
return "Positive"
elif analysis.sentiment.polarity < -0.2:
return "Negative"
else:
return "Neutral"
Step 3: Apply the Function to the DataFrame
Use the pandas.apply
method to classify each review based on its sentiment.
# Apply sentiment classification to the 'Review' column
df['Sentiment'] = df['Review'].apply(classify_sentiment)
# Display the updated DataFrame
print(df)
Sentiment Analysis
Review | Sentiment |
---|---|
The product is fantastic, I love it! | Positive |
Terrible experience, I will not buy again. | Negative |
It's okay, but could be better. | Neutral |
Explanation of the Workflow
- Custom Function: The
classify_sentiment
function uses TextBlob to calculate the sentiment polarity:
- Polarity > 0.2: Positive sentiment
- Polarity < -0.2: Negative sentiment
- Otherwise: Neutral sentiment
- Apply Function: The
apply
method applies theclassify_sentiment
function to each review in theReview
column, efficiently processing each row.
Why Use pandas.apply?
- Flexibility: Allows custom logic for specific data transformations.
- Scalability: Handles large datasets effectively for row- or column-wise operations.
- Ease of Use: Simplifies applying NLP models or custom functions.
Conclusion
Using pandas.apply is a simple and flexible way to perform sentiment analysis and other custom row- or column-based transformations. While it is versatile, keep in mind that vectorized alternatives may offer better performance in some cases. For sentiment analysis, TextBlob is an excellent choice for basic tasks, but for more advanced use cases, libraries like NLTK or spaCy might be more suitable.
For further learning, refer to the pandas documentation and the TextBlob documentation.
Table of Contents
Take a Taste of Easy Scraping!
Get started now!
Step up your web scraping
Find more insights here
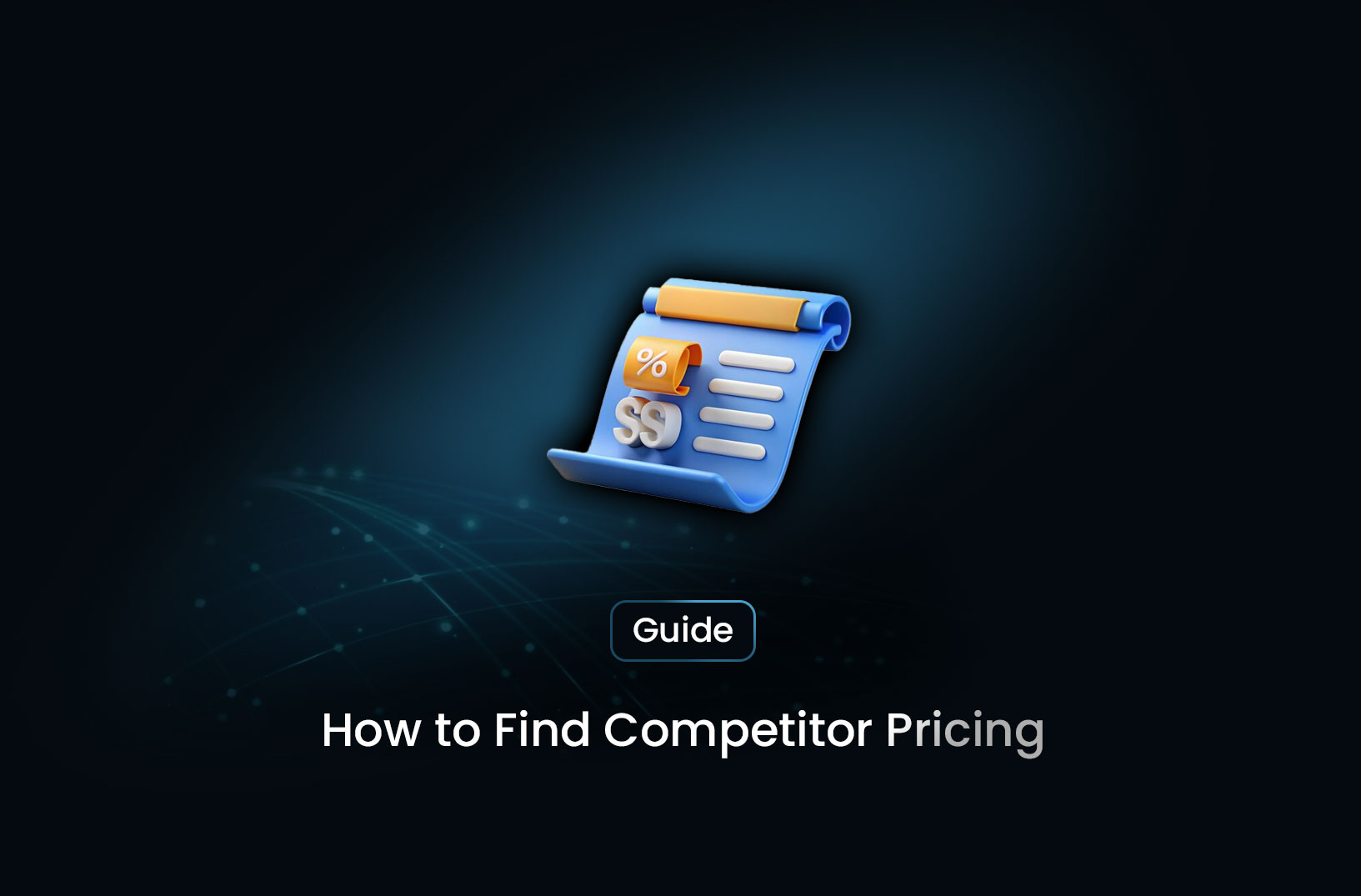
How to Find Competitor Pricing: a Comprehensive Guide
Discover the best methods to track and analyze competitor pricing using web scraping and other market intelligence strategies.
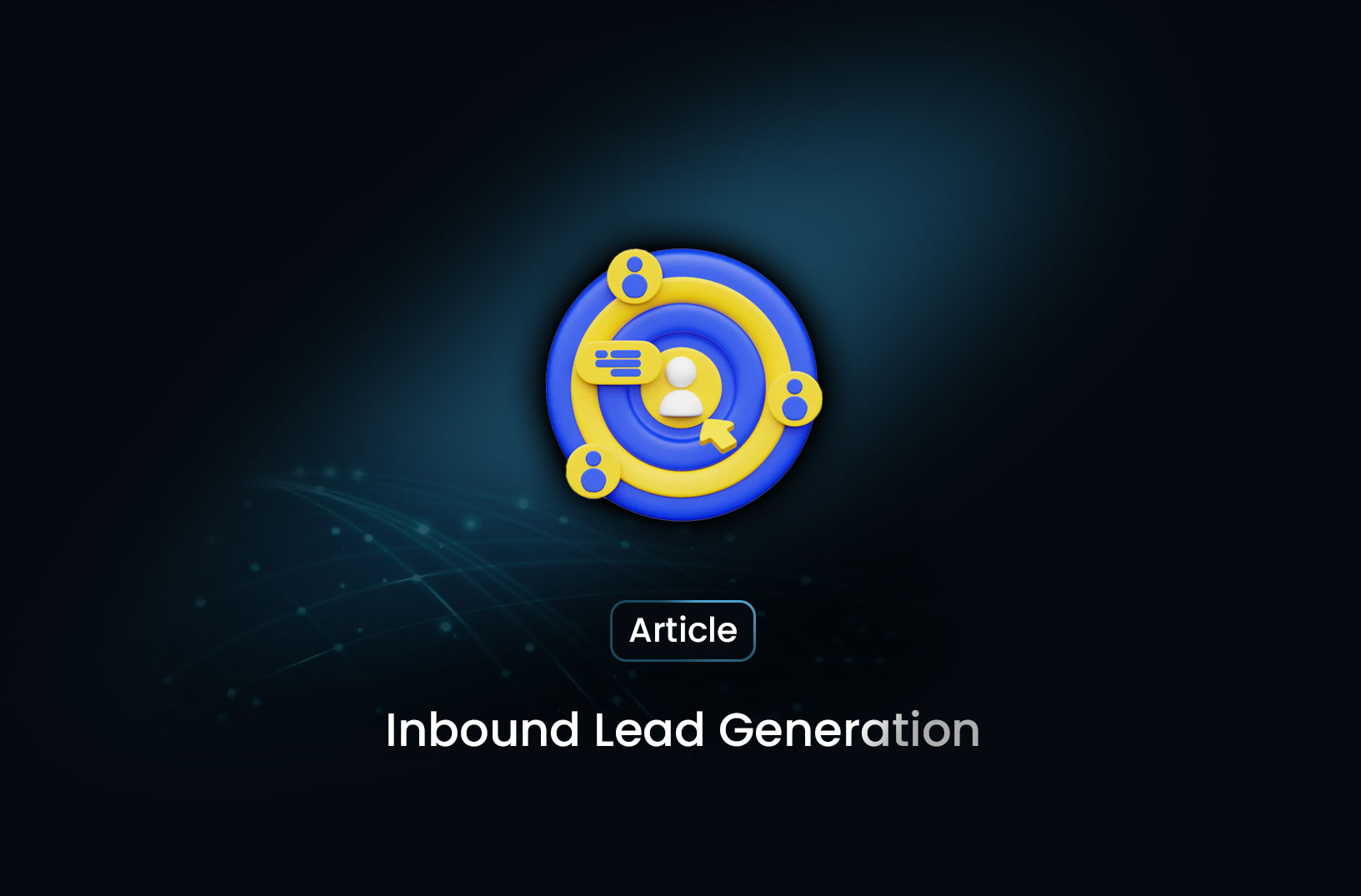
Inbound Lead Generation: A Complete Guide for Businesses
Inbound lead generation is the process of attracting potential customers through content marketing, SEO, and organic engagement.
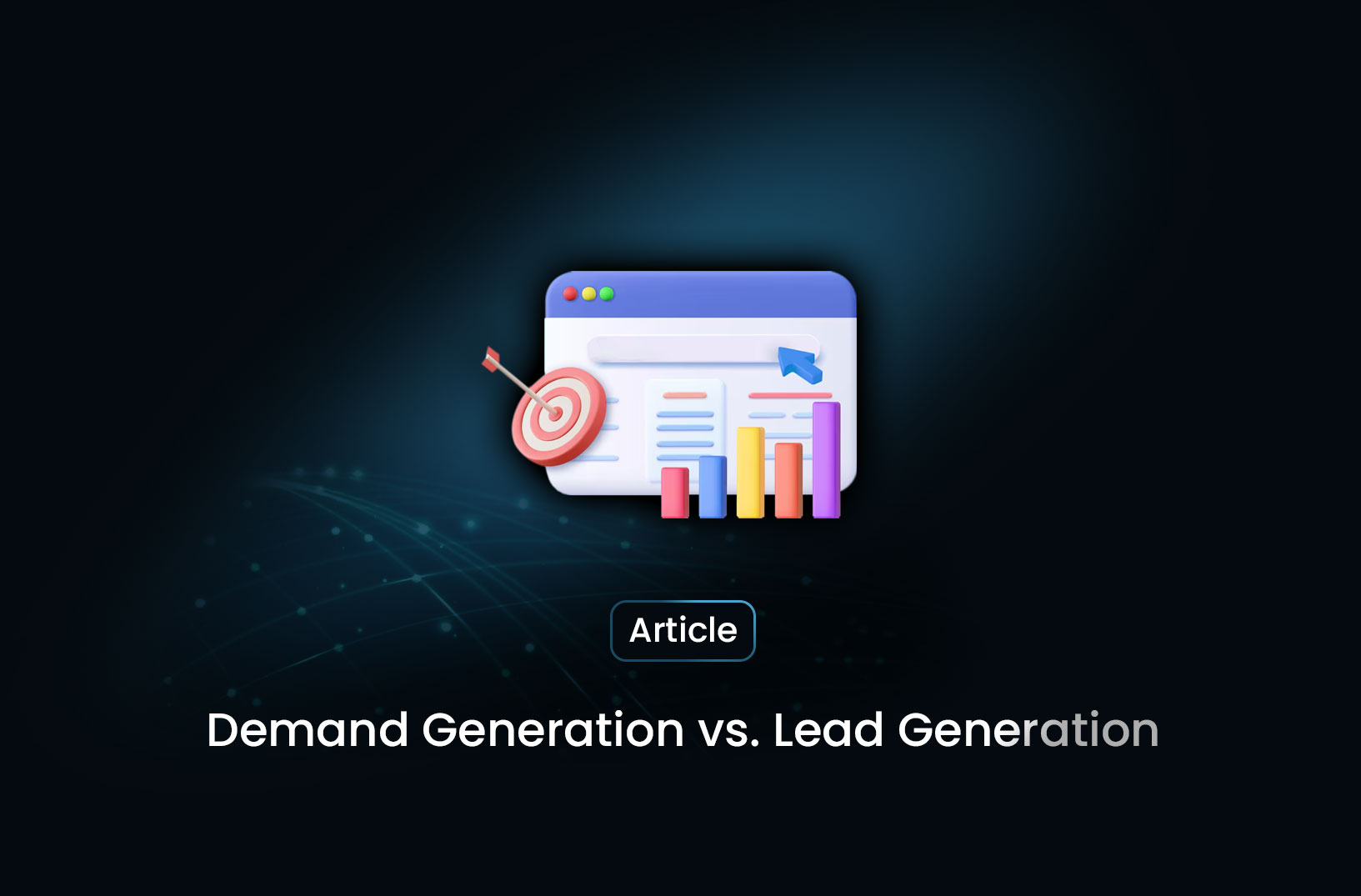
Demand Generation vs. Lead Generation: Key Differences and Strategies
Discover the key differences between demand generation and lead generation—and how web scraping with MrScraper can boost both strategies effectively.
@MrScraper_
@MrScraper