Python Cache Optimization for Faster Data Access
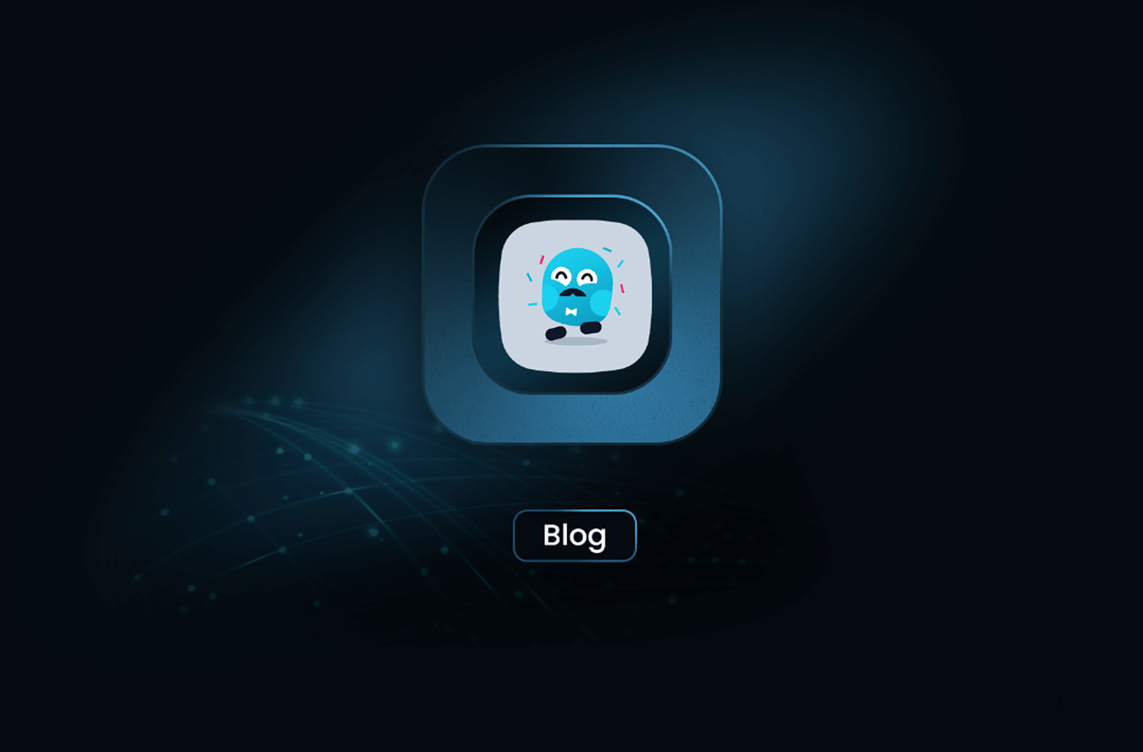
In today's data-driven world, speed is everything. Caching in Python can dramatically improve your data retrieval performance. Let's explore how it works and why it's essential for building efficient applications.
What is Caching?
Caching is a technique used to store frequently accessed data in a temporary storage area called a cache. The primary goal of caching is to reduce the time it takes to access data, thus improving the overall performance of an application. Instead of fetching the data from its original source each time it's needed, caching allows you to retrieve it from the cache, which is much faster.
Why is Caching Important in Python?
Python is a versatile language used in various applications, from web development to data analysis. However, like any other programming language, Python can experience performance bottlenecks, especially when dealing with large datasets or repetitive data access operations. Caching helps mitigate these bottlenecks by reducing the time required to fetch data.
Here are some key benefits of caching in Python:
- Reduced Latency: By storing frequently accessed data in the cache, you can reduce the time it takes to retrieve that data, leading to faster response times.
- Lowered Server Load: Caching reduces the need to repeatedly access the database or external APIs, thereby lowering the load on your servers.
- Improved User Experience: Faster data retrieval results in a smoother and more responsive user experience, which is crucial for retaining users.
How to Implement Caching in Python
There are several ways to implement caching in Python, depending on the specific needs of your application. Here, we'll explore two common methods: using a manual decorator and leveraging third-party caching libraries.
Method 1: Python Caching Using a Manual Decorator
Decorators in Python are a powerful tool that allows you to modify the behavior of a function without changing its source code. One common use case for decorators is to implement caching.
Let's start by creating a simple function that fetches data from a URL:
python
import requests
def get_data(url):
response = requests.get(url)
return response.text
Now, let's create a decorator to cache the results of this function:
python
def cache_decorator(func):
cache = {}
def wrapper(*args):
if args in cache:
return cache[args]
else:
result = func(*args)
cache[args] = result
return result
return wrapper
You can now use this decorator to cache the results of the get_data
function:
python
@cache_decorator
def get_data_cached(url):
return get_data(url)
This simple caching mechanism will store the result of the get_data
function in a dictionary, using the function's arguments as the key. If the function is called again with the same arguments, the cached result is returned instead of making another HTTP request.
Method 2: Using Third-Party Caching Libraries
While manual caching is effective for simple use cases, third-party libraries offer more advanced caching capabilities with minimal effort. One such library is functools
, which provides a built-in caching decorator called lru_cache
.
Here's how you can use lru_cache
to cache the results of the get_data
function:
python
from functools import lru_cache
import requests
@lru_cache(maxsize=100)
def get_data_lru(url):
response = requests.get(url)
return response.text
The lru_cache
decorator caches the results of the get_data_lru
function, allowing you to specify the maximum size of the cache. Once the cache reaches this limit, the least recently used items are removed to make room for new ones.
Best Practices for Python Caching
Caching is a powerful tool, but it's essential to use it wisely. Here are some best practices to keep in mind:
- Cache Appropriate Data: Not all data is suitable for caching. Cache only data that is frequently accessed and doesn't change often.
- Set Cache Expiry: Ensure that cached data is periodically refreshed or invalidated to avoid serving stale data to users.
- Monitor Cache Performance: Regularly monitor the performance of your caching strategy to ensure it's providing the desired benefits.
Conclusion
Implementing cache optimization in Python is a powerful strategy to significantly enhance the performance of your applications, particularly when dealing with repetitive data retrieval tasks. By reducing the time it takes to access frequently requested data, caching not only speeds up your workflows but also reduces the load on your servers.
For those of you who are interested in further optimizing your web scraping processes, you might find my previous post, "Converting cURL Commands to Python for Efficient Web Scraping," to be particularly helpful. Combining the techniques discussed there with effective caching strategies can lead to even more efficient and powerful scraping tools.
Table of Contents
Take a Taste of Easy Scraping!
Get started now!
Step up your web scraping
Find more insights here
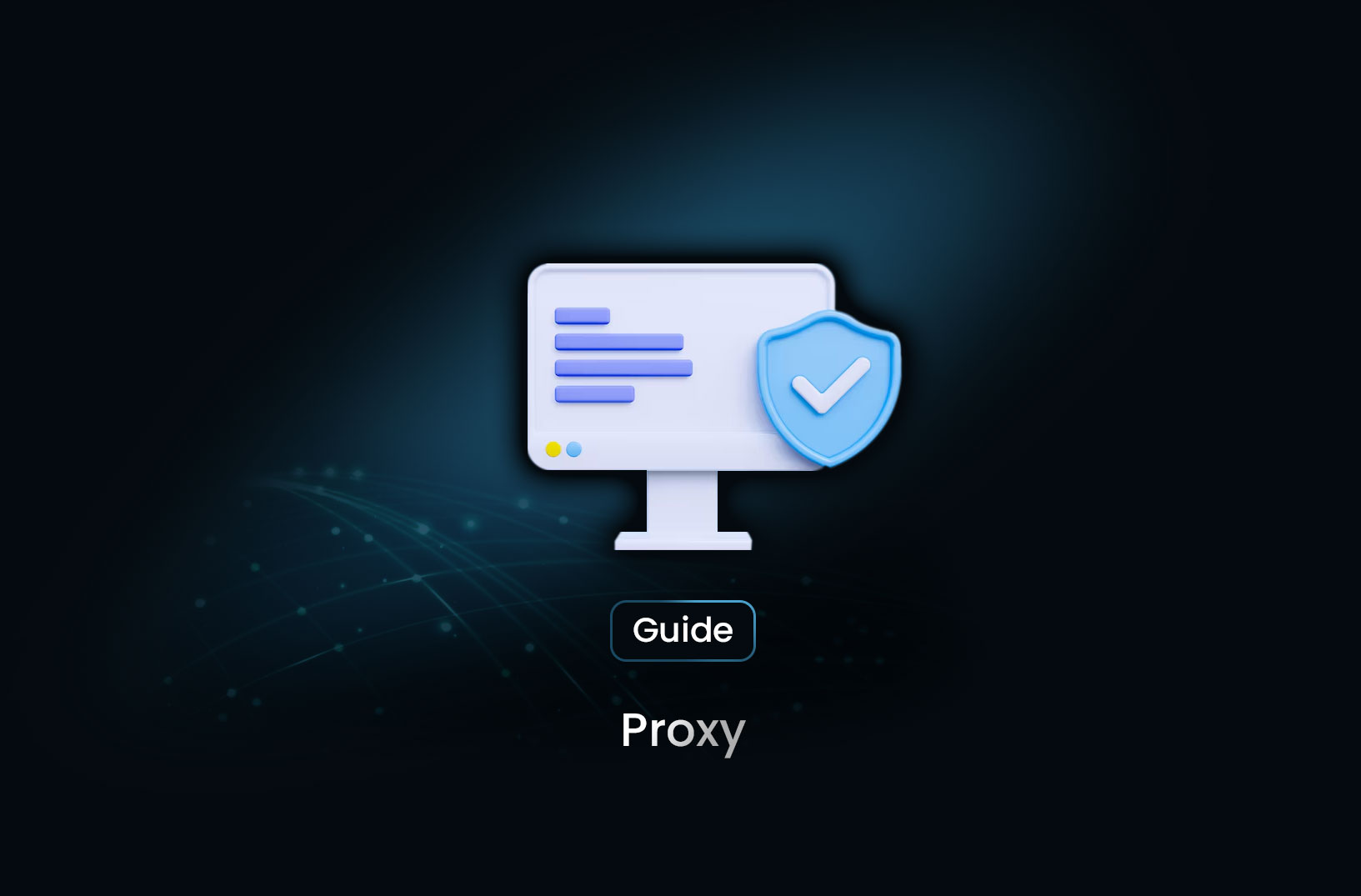
Detecting and Avoiding Proxy Blacklists When Scraping
Learn how to detect and avoid proxy blacklists when web scraping. Identify blacklisted proxies using HTTP codes, CAPTCHA detection, and blacklist checkers. Use proxy rotation, user-agent spoofing, and CAPTCHA-solving techniques to stay undetected.
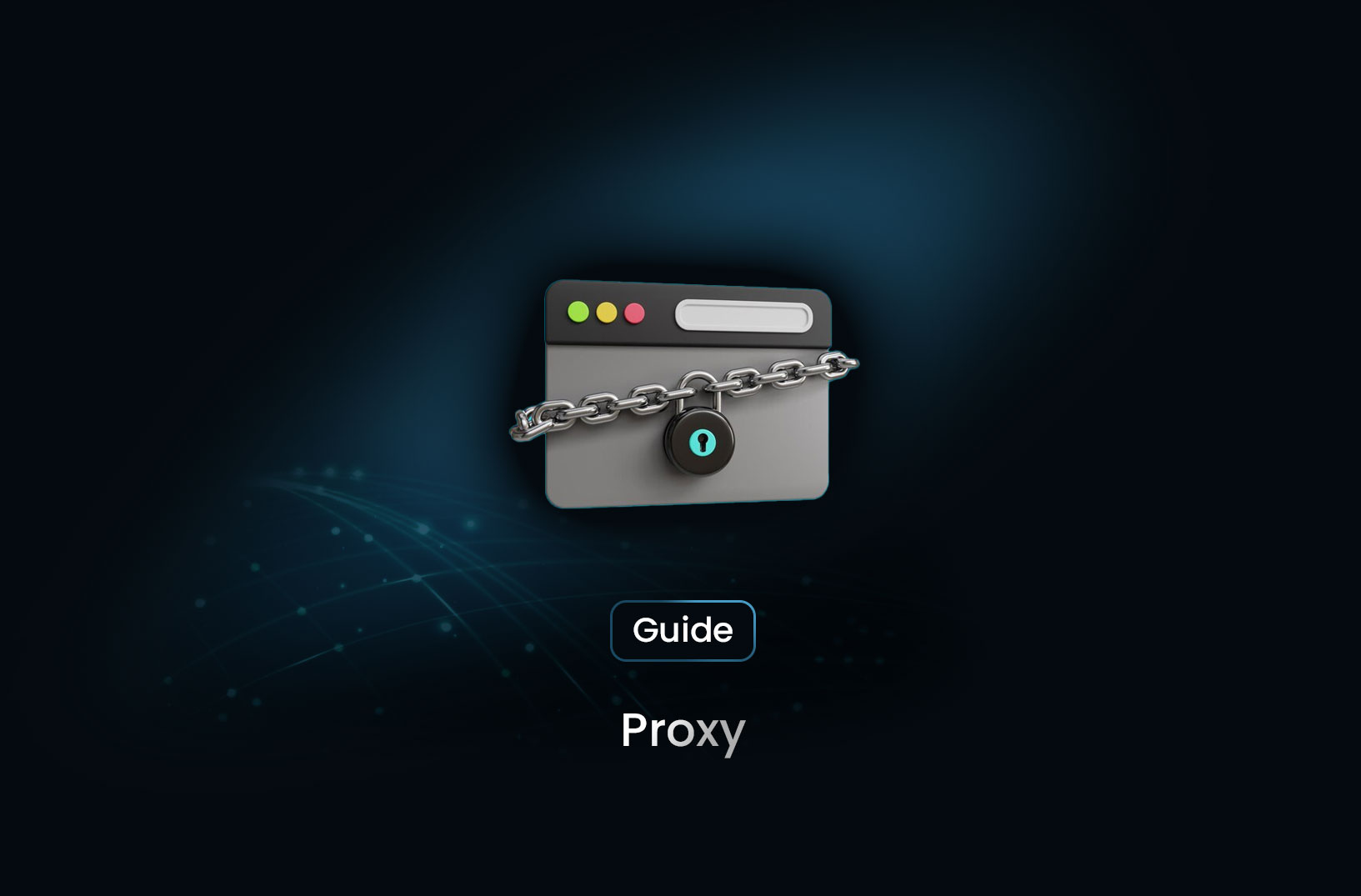
How to Detect if a Website is Blocking Your Proxy
Learn how to detect if a website is blocking your proxy during web scraping. Identify proxy bans using HTTP codes, CAPTCHAs, response delays, and content mismatches. Optimize scraping with rotating proxies, user-agent spoofing, and CAPTCHA-solving techniques.
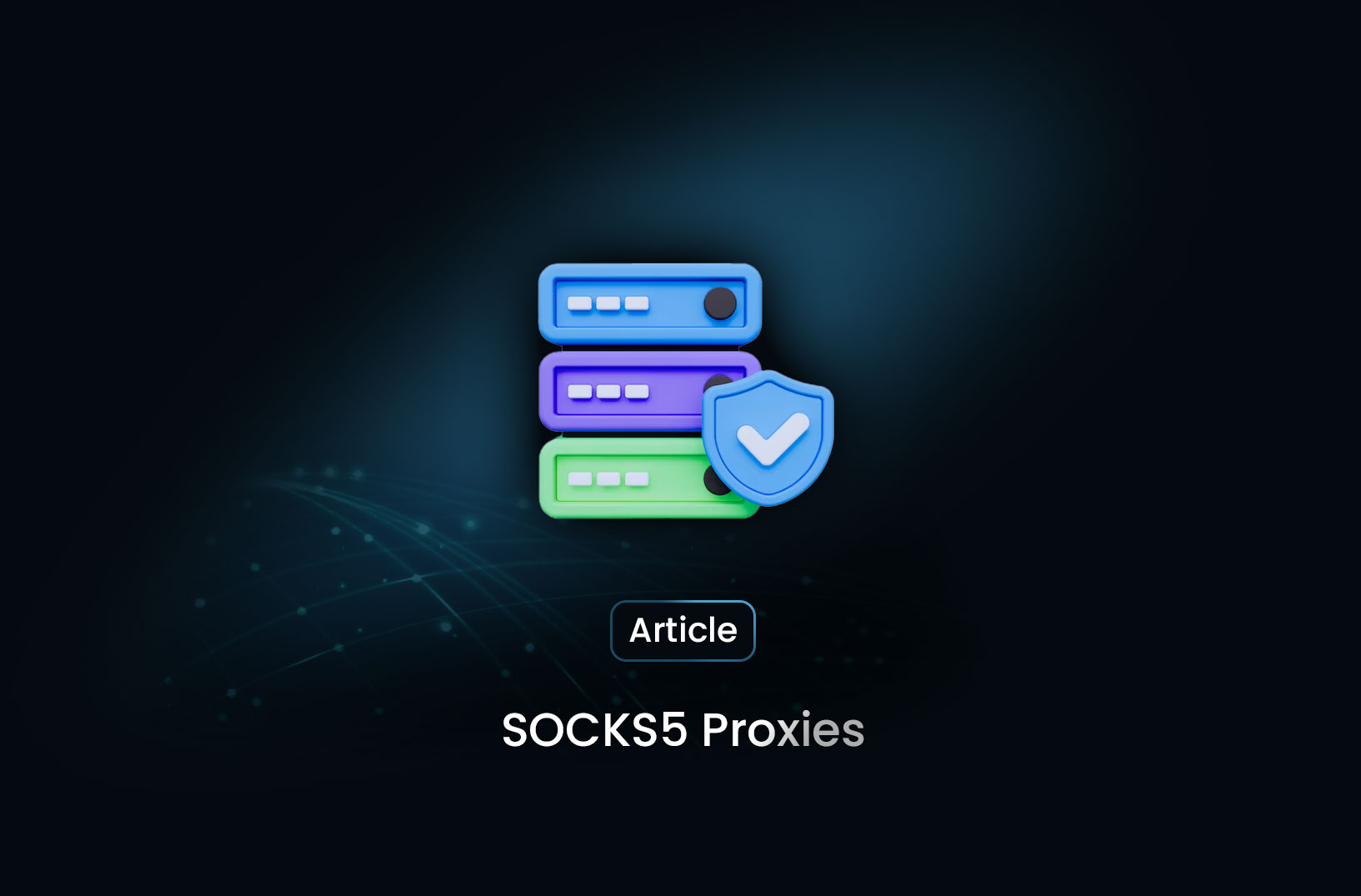
Using SOCKS5 Proxies for Web Scraping
Learn how to use SOCKS5 proxies for web scraping to bypass IP restrictions, enhance security, and extract data efficiently. Discover step-by-step guides, Python code examples, and anti-detection techniques for seamless data scraping.
@MrScraper_
@MrScraper